How Government APIs can address structural racism and inequality
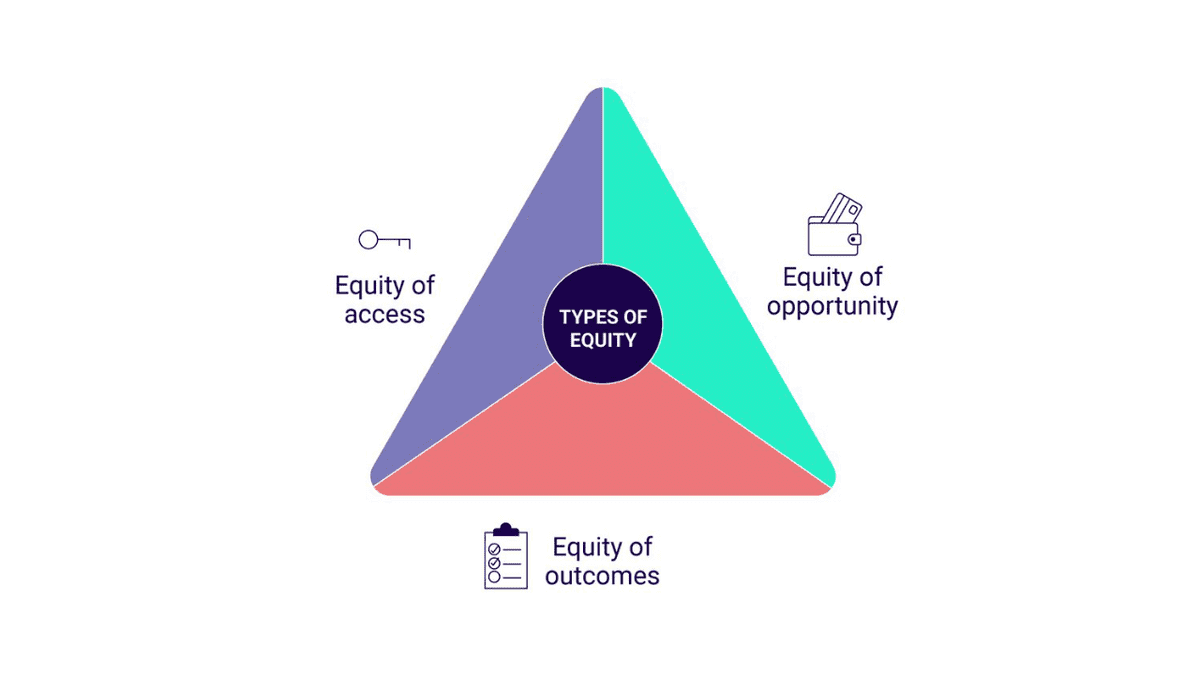
Last updated: 14 August, 2020
The global impacts of COVID-19 have exposed the underlying inequities of our national health systems. This has occurred alongside growing community awareness of the long-term, disproportionate, often state-sanctioned harassment and murder of Black and other non-white citizens by police forces, and the lack of access to essential services many Black, non-white and migrant populations receive. (OK, I realise I risk losing some of you here by stating it as “state-sanctioned murder”, but no one has been charged as yet in Breonna Taylor’s, nor Elijah McClain’s deaths. Breonna was sleeping at home when U.S. cops came in and shot her, Elijah was put in a chokehold because a 911 caller said he was acting suspicious. He became unconscious, and was injected with ketamine by police to revive him. He died of a heart attack.)
Meanwhile, Australia has seen more than 400 deaths of Aboriginal and Torres Strait Islanders die in custody, many incarcerated for traffic offences, move on violations and unpaid fines. And in Europe, a study by the UN’s Working Group of Experts on People of African Descent, found racial profiling by police to be “endemic” in Spain and Germany. They also reported that jails in Italy are disproportionately filled with migrants awaiting trial, who are routinely punished “for less serious crimes than Italians”.
This growing awareness of the structural biases against Black citizens has occurred alongside awareness that other marginalised populations (including Indigenous people, women, migrants, transgender and non-binary people, and gays and lesbians) continue to be excluded from access to services and economic opportunities.
Examples of structural inequality initiated by governments
Here are some examples from recent history that demonstrate that societal structures have specifically excluded access for some citizens:
- In the United States, Minnesota land laws used “racial covenants” to ban non-white people from owning property or applying for mortgages.
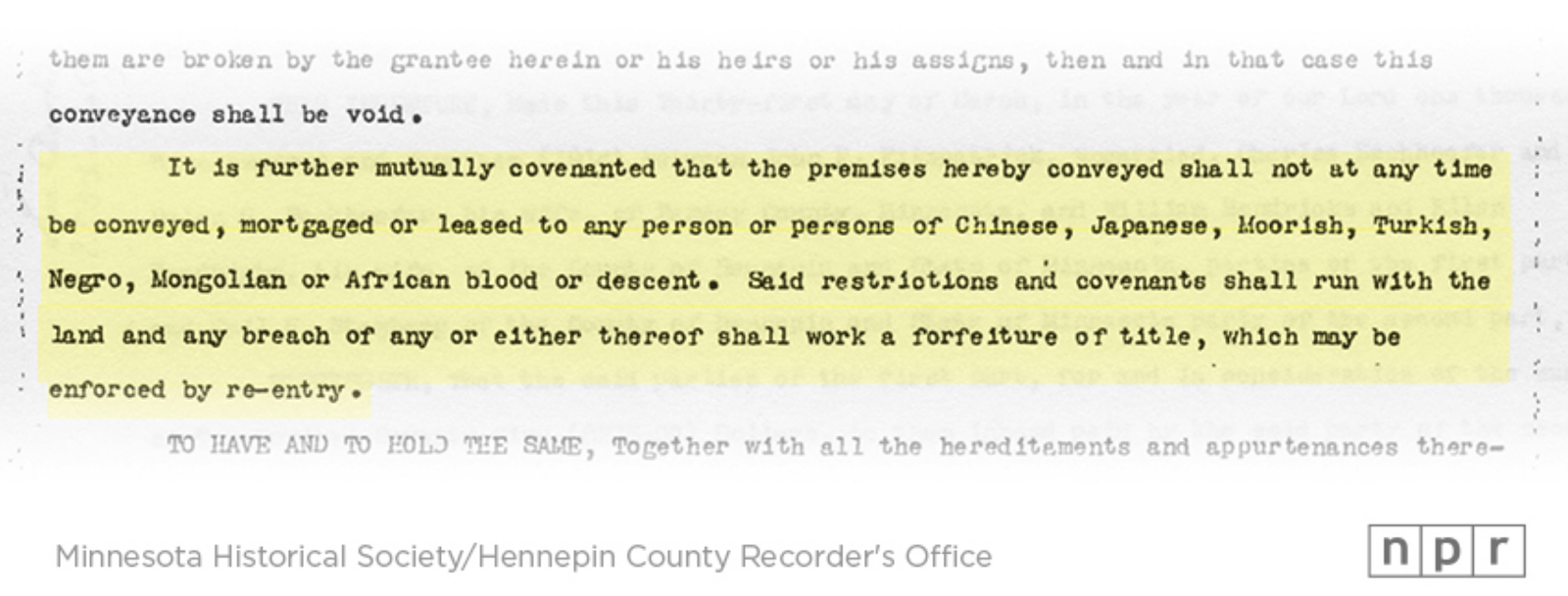
- According to the European Commission, the gender pay gap in the EU stands at 14.8% and has only changed minimally over the last decade. It means that women earn 14.8% on average less per hour than men.
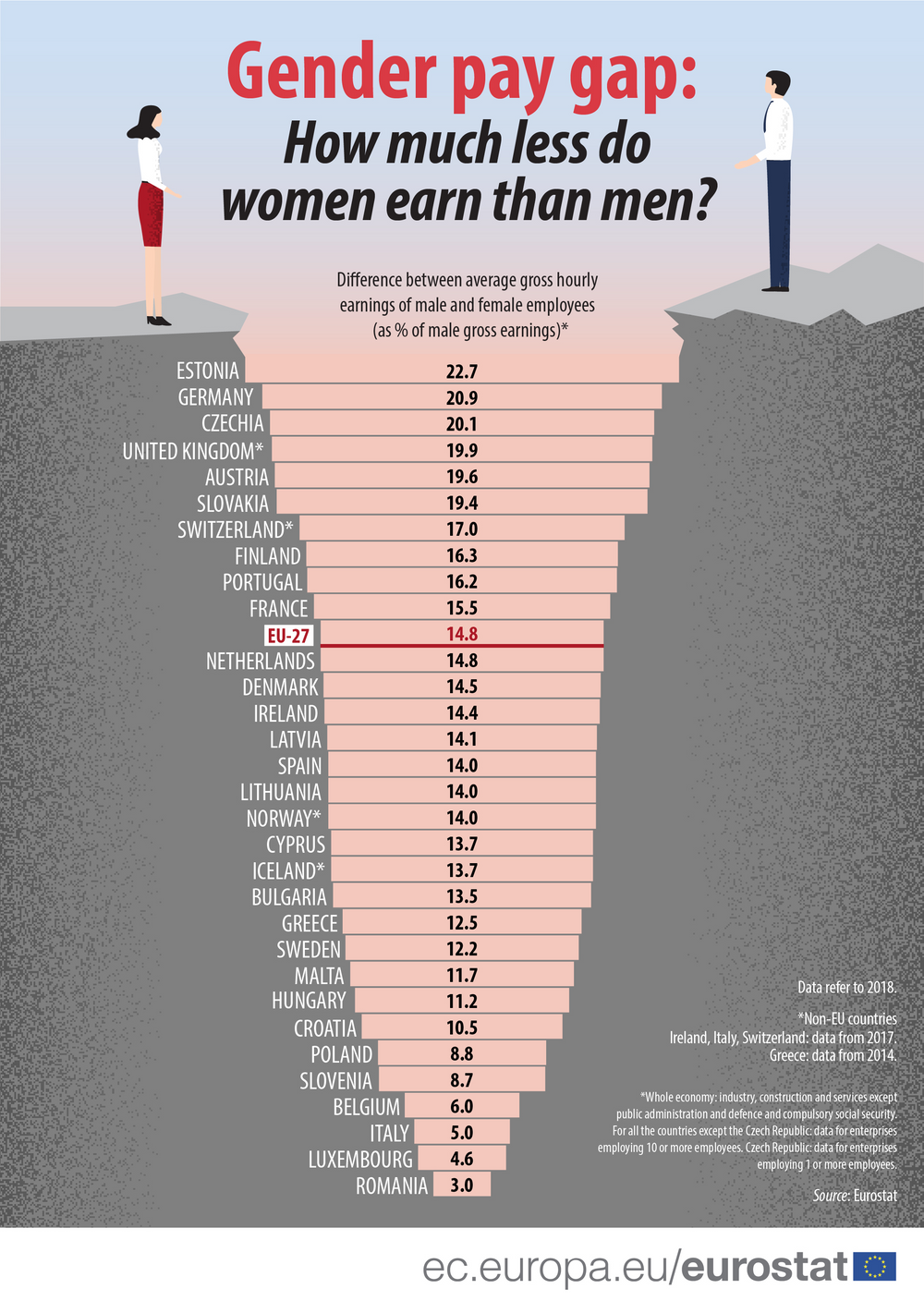
- In Italy, Italian citizenship is only granted automatically to those born to Italian parents. The children of migrants, both those born on Italian soil and those who migrated with their parents as minors, are not automatically granted an Italian passport when they turn 18.
- Globally, over 70 countries criminalise same-sex relationships and/or forms of gender expression. This means also that in these countries not only can you go to jail for loving your partner, it is also legal to discriminate against LGBT people in work, housing and access to services. Again in Italy, for example, draft legislation is only just being debated to criminalise discrimination and hate crimes against LGBT people.
These examples show that the way governments organise and apply laws can structurally deny access to certain population groups. While laws may change and rights improve, because of the long-term historical lack of access, the structural inequality remains. The link to the Minnesota land laws article above, for example, shows that the racial covenants laws continue to define housing access in certain neighborhoods: houses that were granted to white citizens under the laws years ago have since been handed down to generations in white communities, which still accounts for major disparities in the population mix of neighborhoods today. If gender pay and workforce relations are equalised today, there will still be many more men in management roles who took on those roles in the past and who will be unwilling to share management opportunities with women who they see as not having the experience to manage (because they were denied those opportunities in the past).
So, we have shown that governments have enacted laws that generate structural inequalities. And those structural inequalities continue to exist today, even if governments are addressing and changing the historical laws. So we can say governments have helped create societies that are unequal and they need to do something about it.
The move towards digital government and use of APIs
The process of “digital transformation” is currently occurring within many governments. This may start with moving from PDF and paper-based application processes to online and mobile engagement, but extends into using data to plan and provide services, to creating reusable digital components that can be used by non-government partners to build new products, the use of sensors to measure machinery and people movement, and the use of new platforms to engage with businesses and citizens, consult and even allow voting.
Application Programming Interfaces (APIs) are crucial to this digital government evolution, as APIs are technology connectors that link systems and create reusable components that can be used to build a vast array of services. For example, in many countries, citizens are given a secure identity number that they use online to lodge taxes, submit grants, access marriage certificates, apply for services, register education, and so forth.
As governments move towards digital services in which citizens can do all of those things online (or on their mobile), each government department can use an “identity verification API” when they are building their online application forms. This would mean that each department does not have to create the software code to verify the citizen or business identity each time they interact. They can use the one API that all government departments use. Other APIs can be used to connect the citizen’s application form data into a secure database so that the next time the citizen or business connects there is a record of the discussion, even if applications were submitted across multiple departments. (It can also be secured in a way where each government department cannot see what the other department engagement was about, if necessary.)
Government data is also more accessible when it is provided via API. If we look at the way governments around the world share public transport or weather data, ok, sure, they can upload spreadsheets daily of data but that is not useful for realtime apps that help you with trip planning or let you check the weather now. For that data, governments need to create data APIs which allow app developers to connect to real-time data feeds so that when you open an app, you can be confident that the data on the next available train arrival is true, or that the probability of rain in the next two hours is accurate.
How structural inequalities are repeated and reintroduced into digital government
So as we move into digital government processes, the risk is also that we migrate those structural inequalities that governments have helped generate into new digital systems. (It is interesting to note in the gender pay graph above, Estonia, which is heralded for its uptake of digital government processes and government API-enabled infrastructure, has the widest gender pay gap across European countries. So the move to digital government alone will not improve existing social inequities.)
We can see this most easily with artificial intelligence and machine learning systems, particularly in the area of facial recognition. As the data points above show, there is racial bias in policing. If artificial intelligence is creating datasets using existing criminal records, they will have an abundance of Black and other nonwhite faces to include in their machine learning programs. Then if they look at other historical datasets, for example, of political representation, they will add predominantly white faces, as historically, Black or nonwhite citizens were not able to vote, then when they could, they were too busy being denied housing and access to other services or were denied citizenship to be able to participate in political processes at the same level as white men.
So imagine now putting all of that data into a database and then comparing a new face to the dataset. If it is a white face, it will probably look for a match amongst politicians, as that is the data it has more of. If it is a nonwhite face, it is more likely to think there is a higher probability that the face is criminal. This is a simplified example, but aims to show how you could get into the real life situation where Amazon’s facial recognition software (which has been used by governments and police forces) is more likely to match Black members of US Congress to photos of people arrested for crimes.
It is important to note that these large datasets that are fed into the machine learning algorithms are connected via APIs. In fact, if you look at the Electronic Frontier Foundation’s collection of technologies used in U.S. police surveillance, the majority of these require government APIs to be built and in production use before many of the technologies can work.
Planning of services by using historical data accessed via APIs can also further create inequalities. In cities around the world, bike rental programs are being introduced by municipalities. But in New York, for example, the downtown, predominantly white areas of the city had the bike stations set up first. I lived in the Black neighborhood of Harlem last year, and in 2019, Citibike bike stations were only just being introduced. (Citibike was first introduced into New York City in May 2013.) There are still no stations in predominantly non-white areas like Inwood in Manhattan’s north nor in outer, low income neighborhood areas of Brooklyn. So that is a structural example of inequality: the city prioritised allocation of Citibike services to higher income, whiter neighborhoods.
But now, the data on bike usage rates is collected via API, and that data is available to be used to feed into planning systems in order to identify community needs. The bike data is helping inform where new services should be located or to measure a level of dynamism in residential mobility activity that would indicate to city planners that they should provide more services and amenity in those areas. What if city planners used this bike usage data (analysed via the API feed) to decide where to place public toilets or supermarkets? There is no initial underlying consideration by decision-makers as to whether the API data feed is biased before connecting it up to make planning and amenity decisions. The webpage describing how to access the real time data feed does not specifically mention the potential inequity baked into the data because of how Citibike services have been historically distributed in New York City. So there are no notes of caution for potential users of the API feed before they go on to build new products and services based off the data.
So as we move current city government systems into digital processes, you can see how we can again replicate and further entrench structural inequality.
I want to give one more example. Around the world, there is an API standard called “Open311”. All city government systems have a form of citizen complaint hotline where people can report graffiti, vandalism to city infrastructure like bus stops, potholes, noise complaints and so on. Using APIs, the Open311 system first takes the call (or email, or mobile app report, or in-office attendance, or however else the citizen or business reports the complaint). Their issue is then fed (via API) to the asset maintenance department or wherever responds to it. The issue is added to the asset maintenance works roster, the work is remediated, and when completed, the Open311 API routes the outcome back to the citizen informing them of the resolution of the issue.
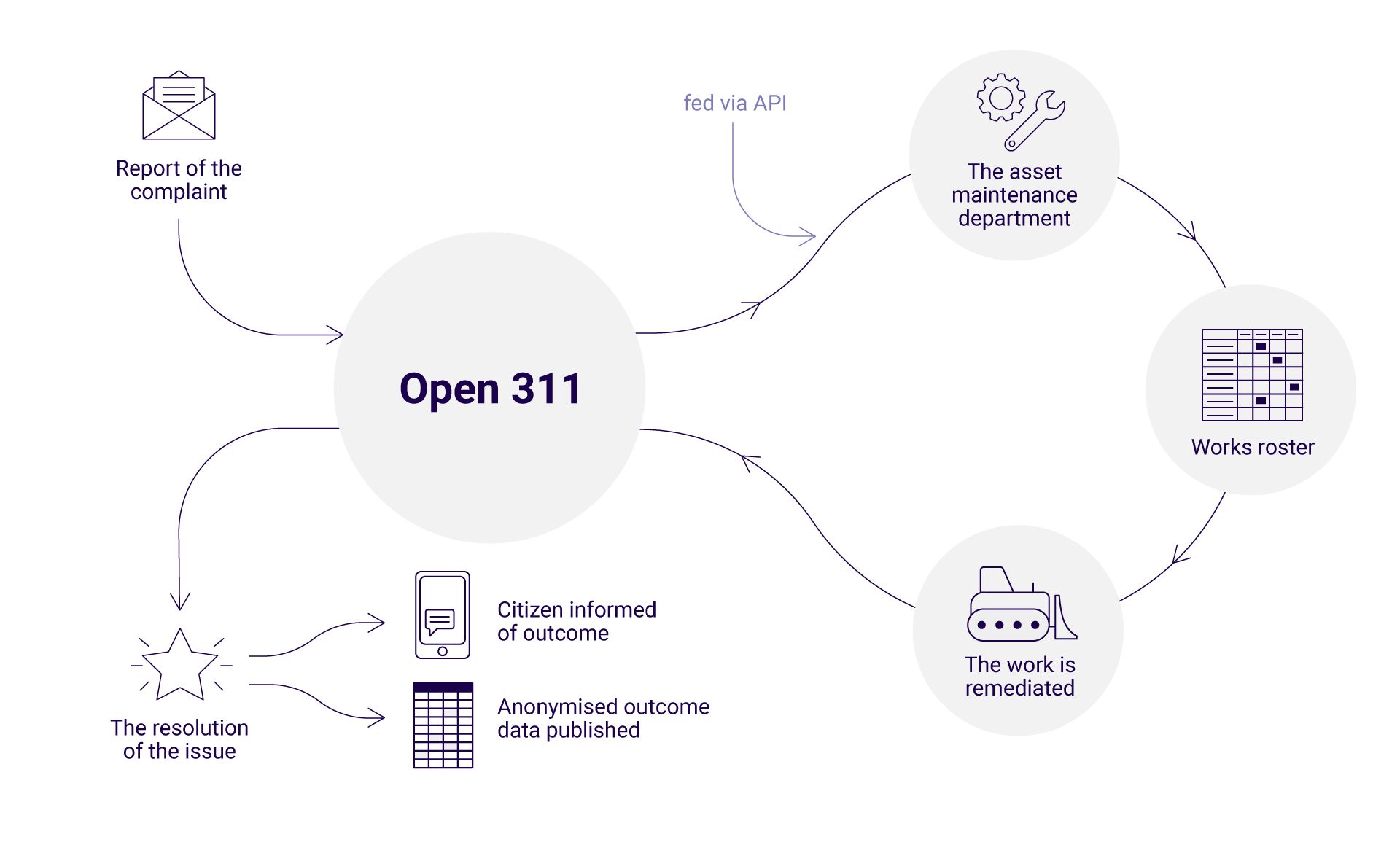
Under Open311, identifying details of the complainant and anything that might be related to their specific address is removed and the rest of the data is released as open data so that there is a degree of transparency in how city governments are responding to citizen complaints. But residents in higher income neighborhoods may feel more engaged with the city government, understand the services available to them, and have the free time and resources to make the call to the city. People in low income areas might not know this service exists, they might work shift work or are juggling family caring duties or be in low income, physically demanding work which leaves them tired, they may live in rental housing and not feel a sense of ownership in their community. So they may make less calls to the Open311 system. If city governments are allocating asset maintenance based on Open311 calls, then you can see in this example how, over time, more resources may end up being directed to higher income neighborhoods, further widening inequality in city allocation of resources.
The challenge of discussing equity in digital government API activities
One of the pieces of work I am most proud of in my life is my recent work contributing to the development of an API Framework for Digital Government. This study, which I co-authored with the European Commission’s Joint Research Centre, reviewed over 350 best practice documents from around the globe to create a cohesive API framework consisting of 12 proposals.
The Framework aims to encourage governments to shift from building one-off departmental-based APIs to integrating an API approach across all of government digital activity. The reason why I am most proud of this work is because it is one of the first technology papers that actively discusses the need for equity-based approaches to be embedded in government’s API technological practices and organisational processes. In the past, equity has been considered more in terms of the “digital divide”. So, for example, if we move digital services online, do senior citizens or rural residents have the computer literacy, internet bandwidth and available technology devices (laptop or smartphone) to allow them to access the service?
But this project, and a related study into the costs, benefits and current landscape of government APIs around the globe, are two of the first government API-related documents that specifically discuss the potential equity impacts of APIs when being implemented by the government.
In my personal opinion, it was challenging to include this discussion for two reasons:
- There is a lack of evidence of the equity impacts of government APIs
- There is a norm amongst policy makers and technologists that equity considerations belong in “other” documents, such as in broader policy documents and do not have a place in technology-specific policy.
I came to these conclusions through two activities related to researching and when collaborating to the team that drafted the API framework:
- A global literature review of best practice documents that surveyed over 350 documents from the past four years, most specifically dealing with government APIs and some from leading industry API implementations
- Four workshops held with government API stakeholders, with several activities specifically delivered to discuss equity impacts.
Lack of literature
The challenge when co-authoring the Digital Government API Framework was that it was intended as an evidence-based approach. How could I include equity considerations in the Framework if there was no evidence that inequality was being generated from digital government APIs? Our methodology focused on identifying some of the gaps, and the broader study work discussed some of the potential equity impacts that logically could flow from API implementations (such as the description of the Open311 case given above).
But what we all (as government API practitioners) need, going forward, is that all government API studies include an equity dimension. For example, in a followup study that I am now conducting to look at how government APIs can be discoverable by potential users, we need to include an equity lens that measures:
- Are Government API catalogues and websites using web accessibility standards to ensure they are accessible by developers of all abilities? This is a European Commission requirement, but Tatiana Mac, who has helped create a number of checklists and resources to implement accessibility guidelines in tech describes it best:
- Are Government APIs accessible by small businesses and startups without barriers to market entry in the same way they are accessible to large corporations? (This is an acknowledged issue in the European Commission’s EU Data Strategy which notes that there is a risk that data is made available in a manner that reinforces imbalances of power in the market.)
Ideally, we would have business data available that was good enough to also look at the makeup of the startups, small businesses and corporations that are using the government APIs, to see if minority-based businesses have access (for example, the number of businesses located in low income neighborhoods, or those with women or non-white participation in leadership teams).
And even more ideally, we would then go on to measure the value being generated from government APIs and see if that is equitably distributed across society (the ability to measure the value of API ecosystems is the longer term mission and work of Platformable). For example, if government identity APIs are used to help citizens speed up opening business bank accounts, are migrant-owned businesses using them as much as white, male-owned businesses, on a proportional basis (i.e. are APIs enabling equality of access)? And then, when they are using them, do they get as much access to loans as their white business counterparts (i.e. are the APIs generating the equality of opportunity that leads to equitable outcomes?).
But those two ideal metrics are a tough ask at the moment because:
- There is not good enough disaggregated data on the composition of registered businesses in Europe. (For example, Europe’s main small and medium enterprise datasets including the Structural Business Statistics and the annual survey on the access to finance of enterprises do not provide data on ownership rates and make up of businesses from a gender, disability, race or migrant-owned basis.)
- There are not enough models that measure the value flow being generated by digital government APIs (Yes! That was also a finding of both the study and the Framework, and the Framework includes proposals to address this gap.)
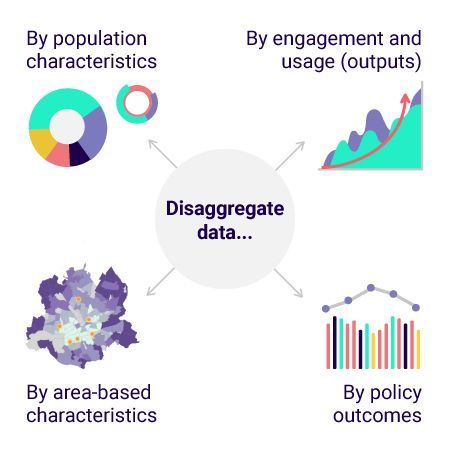
Norms amongst technologists not to discuss equity impacts
During the government API framework project workshops , I wondered if some participants did not feel comfortable with discussions that focused on equity. Part of this hesitancy was my fault and reflects the same issues I had when I worked in health inequalities policy research in Australia, so I should have known better. When you talk about inequitable impacts of policies, people immediately start questioning the proxy you are using to show uneven outcomes on specific populations as there are multiple causal pathways. So for example, if you use low income populations and measure the impacts on low income, people argue that income inequality is a separate issue to digital engagement. In Europe, you can’t really discuss race because there isn’t the data available, and as some decision-makers and leaders have pointed out, culturally (in countries like France), race discussions can be seen as “vulgar” interpretations of citizen population groupings. (Apparently the French are more comfortable describing inequities as being income-driven, as though race does not come into that, an opinion noted scholar and author Professor Crystal Fleming rejects outright.) In the end, we had to limit our discussions to the "digital divide", which didn’t fully encompass all of the ways that inequality and structural disadvantage can be reinforced through government implementation of APIs (but again, without the evidence related to APIs, it was difficult to discuss the other potential impacts).
While reducing inequality is recognised in the higher level European policy documents such as Europe 2030, which describes the European Union’s commitment to Sustainable Development Goals, there is a sense amongst some technologists that discussing equity in technology policy documents is overtly “political” and should be avoided. There is a sense that technologists do not feel equipped enough to participate in the discussion, and that it should be left to broader policy makers.
When describing cases like the facial recognition example given above, some API technologists argue that the appropriate place for the equity discussion then is in the artificial intelligence policies and not in the API work. The same argument is made about data collection: equity perhaps should be covered in the data policies and not in the API framework, which after all, is a mediating (connector) technology between two other systems. My personal take is that it is better to have the equity discussion twice rather than not at all. So if it is discussed in the data or AI policy implementation as well as in the API implementation discussions, then we have a chance of addressing equity more than if it is assumed that some other policy implementation area will address and plan for potential adverse impacts. (Also, it is the API that is enabling the data to be fed into machine learning so it is the first opportunity to reflect: are we reinforcing inequity at this stage, before it becomes an issue for the algorithm makers. Maybe at the API stage we can set rules around how the API should be used in equitable ways to help guide the AI implementations.)
In addition to the reluctance I witnessed at the workshops, I have seen other technologists also show discomfort with this discussion. Whenever I have been at a smart city conference where a digital government lead has presented on Open311, I have always raised the issue I discussed above of inadvertently allocating more resources to higher income communities and asked whether they have any suggestions for how to implement Open311 in a way that avoids this potential inequality. Every. Single. Time. I am given the same answer: “That is what is so great about open data, with open data you can do that kind of analysis.” My followup question is always: “Yes, but do you?” And the answer, again, is always the same, “Sorry I have a call coming in I have to take, byeee!” Every. Single. Time.
Other variants of this experience include working with government API leads who are able to show mapping of data but when you ask if they can show population data using socioeconomic indicators (for example, by shading map areas - which is called a choropleth - depending on levels of low income concentration, etc), they all say it is on their work list of priorities and they will do it in future.
I have also pitched articles to government tech media to write about this and have been told it is out of scope.
Yes, I acknowledge in all of these cases, it may be more a reflection on my lack of advocacy skills that has prevented more action on equity in government API circles (wow, maybe I’m not very good at this.). This article is about trying to uncover those obstacles and enlist you all as co-partners in finding new ways to overcome these barriers.
Just to be clear, I am not blaming the technologists for what I perceive as their lack of willingness to incorporate equity discussions. As the literature review demonstrated, this is not a norm of how technology policy is written. The multiple causal pathways that influence disadvantage and lack of agreed proxy measurement (such as measuring impacts on low income populations or by race/migrant status) also makes technologists hesitant to participate more assertively. (I imagine this article will also face its share of critics who will want to debate the nuance of some of my examples and debate whether inequity is really at the heart of the specific example, rather than taking the time to contemplate other examples where inequity may be cultivated through digital government processes.)
My own experience is that when you do have an opinion or want to address this, you use up a lot of political capital in arguing the case and are often sidelined for being the radical/utopian/non-pragmatic thinker. This can lead to not being invited into implementation discussions, or to having to defend arguments that really prove the link between income and digital government API implementation. So if you are not confident or this is new to you, as a technologist it could bring up a lot of fear or a sense of being out of one’s depth. And like I say, I believe there is a real risk you will be sidelined from participating in future work if you bring this up too often. (For example, I was once invited as an outside member to a group at the Premier’s office in Victoria that was looking at addressing inequity. Using research from the health institute I worked at, I showed that some low-income jobs were worse for your health than being unemployed. The Minister’s key adviser literally scoffed at me when I pointed this out and I was not invited back again.)
Equity goals as performative
I believe we can improve equity and opportunity through the reorientation to digital government processes. But to date, much of this work, I believe, has been largely performative.
I have already given some examples, the responses when I raise Open311 about how amazing it is we have access to this data now, and the “it’s on the list” response for creating tools that allow socioeconomic analysis are examples of a performative responses where nothing ends up getting done.
Unfortunately, I believe the European Union’s references to equity in the Sustainable Development Goals (SDGs)and in the Green Deal are largely performative. The goal of addressing inequity is not mandated to report in lower level policies and there is no guidance on how to incorporate addressing this goal in the various digital government strategies of the European Commission. (I wrote that last sentence about three days before the UN Deputy Secretary-General warned that the world was behind target for achieving the SDGs even before COVID-19 came along.)
There is quite often a lack of the basic data tools available that would allow any equity reporting or analysis. For example, Europe’s COVID-19 data is unable to do any equity analysis other than gender. This is absolutely outrageous. It is hard to locate any data on the level of migrants that work in the healthcare, public transport, hospitality, food delivery, grocery services and personal care industries in Europe. I believe this would follow a global pattern (Europe, UK, US, Singapore to name a few) whereby we are putting migrant workers at greatest risk of exposure to COVID-19 through inadequate PPE and support services in the workplace, poor working conditions, and inadequate income payments. In Europe, we are unable to analyse data on COVID-19 impacts by migrant status or by income level which would expose many of these inequities. There are some studies being done, including on gender impacts, but there are no structural, regularly published datasets available from the European Centre for Disease Prevention and Control.
(Since first publishing this article last night, the Guardian has now reported that in Catalonia, Spain, where I live, an uptick in COVID-19 cases is being liked "to seasonal farm workers – who often work in crowded conditions and at times have been left sleeping rough – despite warnings that these conditions leave them more exposed to the virus." The Guardian article, by Ashifa Kassam, interviews Helena Legido-Quigley, a professor in public health who confirms: “There was no planning at all. This was a health crisis but also a social crisis.”)
Both Our World in Data and Open Government Partnership recommend disaggregating data by population characteristics (and providing in machine readable format, such as APIs) as a best practices in how governments should be publishing COVID-19 testing data. Our World in Data, for example, points to Belgium as an example of good practice. Belgium does provide data by age, sex and region but not by race or migrant-status, despite the European Commission noting in 2018 that, across Europe, “no comprehensive migrant health policy is put in place and the need for tools to ensure accountability and evaluation remains.”
As much as Europe believes we are more democratic and equitable than the US, the US has better data systems on measuring disparities, although for COVID-19 it has been severely lacking and at one point required the New York Times to sue the CDC to get access to disaggregated data to demonstrate the unequal distribution of health outcomes from COVID-19. I was also involved in a public health working group in New York where we advocated for disaggregated data to be made available in both dataset and API format from New York City and New York State. New York City now shows rates of COVID based on race, zip code, and income level. New York State still does not. The European Commission does not have any member state that provides disaggregated data in this format.
All the same, New York City is still largely performative when it comes to addressing equity issues by sharing data. The Mayor has made a big show of painting “Black Lives Matter” but does not provide any data on whether Black residents are benefiting from the city’s reopening strategies. They hired McKinsey (or maybe that was New York State, whatever) who at the time was doing a lot of Twitter promo ads on racial equality using their data like this:
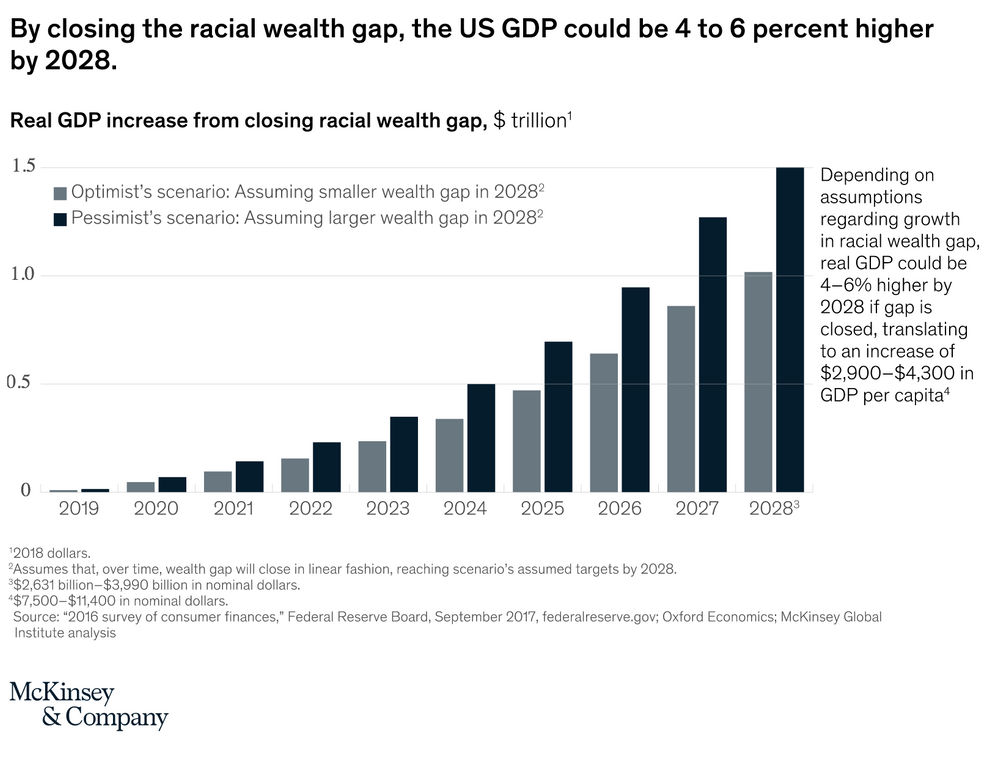
But the lack of disaggregated data on reopening outcomes being shared publicly suggests that either McKinsey didn’t factor in that kind of analysis when guiding New York City and State through their reopening strategies, or were as ineffective when advocating for disaggregated data as I have shown I was when speaking with Open311 or with that Ministerial Group in Australia. (Hey, does that mean I am eligible for a job at McKinsey?)
How to use Government APIs to Address Structural Racism and Inequality
So far, we have shown that governments create and reinforce structural inequality, and that, left unchecked, the move towards digital government processes (often enabled by APIs) will also reinforce that same structural inequality. So what can we do to reduce and eliminate those risks?
OK. So my answer is going to seem a bit naïve, I admit it.
We need to be collecting and reporting disaggregated data at every opportunity. This data needs to be made available via API so that it can be embedded in decision-making processes and evaluation/measurement/monitoring work.
Here’s why it is a bit naïve:
- Making this data available via API suggests that it could be then embedded into data visualization tools like dashboards. However, are dashboards an effective policy tool? If you look at COVID-19 supply chain management and reopening strategies, it does not appear that they are used to guide decision-making around the globe, particularly not in the U.S.
- Other government leaders, many that I respect a lot and who are staunch advocates of open data, also point out that open data will not be the solution to complex structural problems such as police violence and lack of oversight:
While I agree that in and of itself, the disaggregated data will not be enough, it is essential to being able to measure and expose structural inequalities. In my work on health inequalities back in the day, we had a model called the three types of equity:
- Equity of access: Measuring that all people have equal access to the resources and services needed to live a healthy, safe and sustainable life. For example, that childcare services are open and eligible to everyone in a community.
- Equity of opportunity: Measuring that all people have the same structural opportunities to access those services. So for example, that pricing is not a barrier excluding some residents from accessing the childcare, or that childcare is not located in areas inaccessible via public transport, or that the childcare service has a ramp for parents in wheelchairs to enter.
- Equity of outcomes: Measuring that all people are receiving the benefits of the initiative. For example, by measuring the level of childcare service use by specific populations and seeing if that is equal to the proportion of those populations in the local community. (We often stick to measuring outcomes, which is the more immediate value generated for participants, rather than impacts, which is the longer term benefits. In this case, that would be something like “stronger, more resilient families” which has multiple influences and for which it is harder to measure the influence of childcare in creating that impact, yet it undoubtedly does have a role to play.)
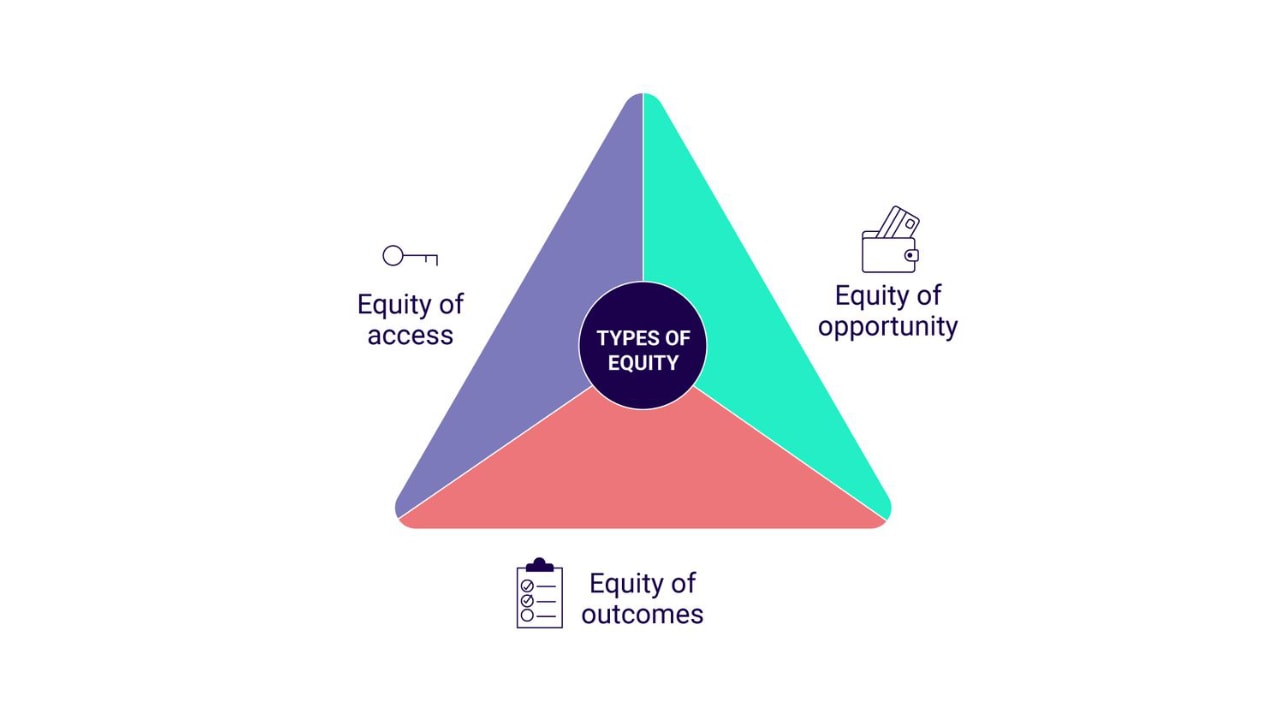
Disaggregated data at least gives advocates a fighting chance. If we want to make the argument that economies improve if all citizens can participate, or that healthcare costs are lower for everyone if those with greatest needs are supported, or that education outcomes are improved if we have diverse schooling environments, we need the data to show that.
And while I recognise that disaggregated data is not the panacea for structural racism, it obviously does have an impact. The Chicago Police Department has an Arrests API which shares anonymized, disaggregated data on arrest trends by race, age, and crime/misdemeanour. It was used by the media to expose inequalities in policing practices which saw over-representation of Black people being arrested. The Chicago Police’s response to this exposure was to shut down the API access.
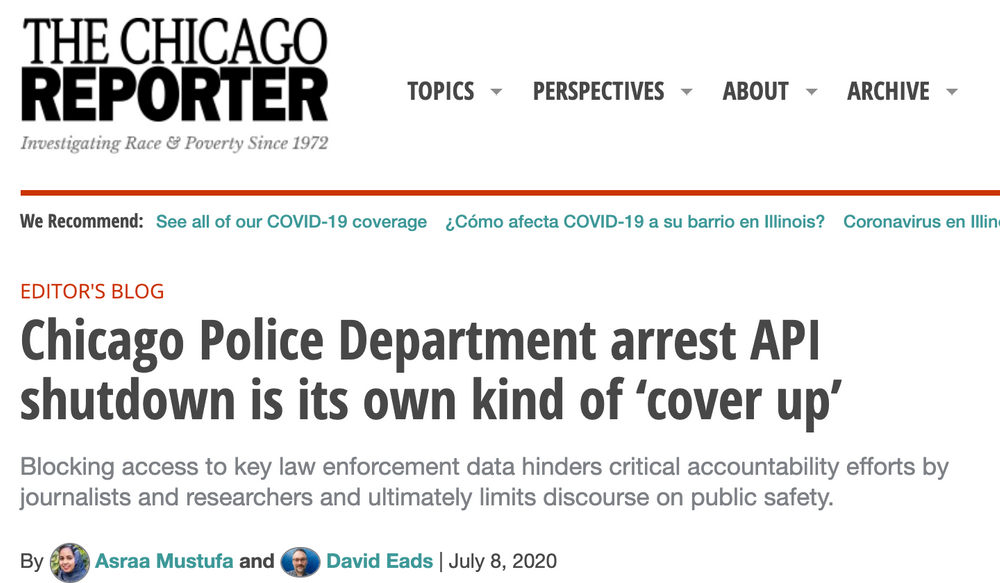
So clearly decision-makers and institutions are afraid of the policy impacts of disaggregated data. (But as Mark Headd pointed out in his tweet thread above, access to this API isn’t enough: he had seen cases where city councillors banned the use of teargas, but then had to still negotiate that ban with the police union. The same issue came up when a city tried to institute a more transparent process on police misconduct. So I’m not saying the disaggregated data APIs are enough to move the needle on structural racism, just that it is an essential building block for designing and delivering equitable digital government.)
As noted above, the US CDC had to be sued to gain access to the disaggregated data that exposed COVID-19 inequalities (Anyone in Europe want to work with me on getting disaggregated data from the European ECDC by the way?). Again, the availability of this data is something that institutions are avoiding in case it has policy implications.
How to use disaggregated data APIs to address structural racism and inequity
If this disaggregated data was available via API, I see it playing two key roles:
1. Embed differential analysis in policy making processes
Governments prepare research documents, background papers, and so on to inform decision-makers on an issue, prior to policy formulation. Researchers and document writers need access to an API so that they can click a button and automatically embed disaggregated data in their reports. This will help expose differential population characteristics that may then be addressed by specific strategies.
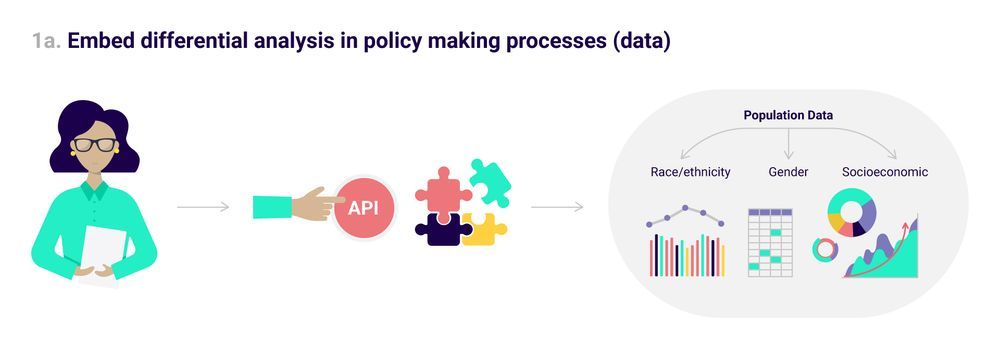
I am a big fan of universal and targeted processes. So for example, if a background report was being written to introduce Open311, it would show the current population makeup across a city, disaggregated by population characteristics like race, ethnicity and income levels. Then when planning implementation, someone could note that while Open311 will be available for everyone (universal), that maybe the city’s fleet would need to supplement this with outreach in areas with higher concentrations of low income neighborhoods (targeted) as possibly those residents would be working more shiftwork or in lower paid jobs which might limit their ability to use their spare time to connect with the city’s services. Or if there was an area with a high concentration of foreign-born residents, they may translate some promotional materials on what Open311 is in relevant languages. The best practice evidence review I conducted on Government APIs did not show any use of universal and targeted strategies. There was an overall sense that only universal (population-wide) strategies are needed.
Policy makers and implementation leaders can also use the API to then click a button to map how their policies may be introduced. For example, when deciding where to locate a new bike rental or childcare service, you would click a button and see where current services are located against a choropleth map of socioeconomic background.
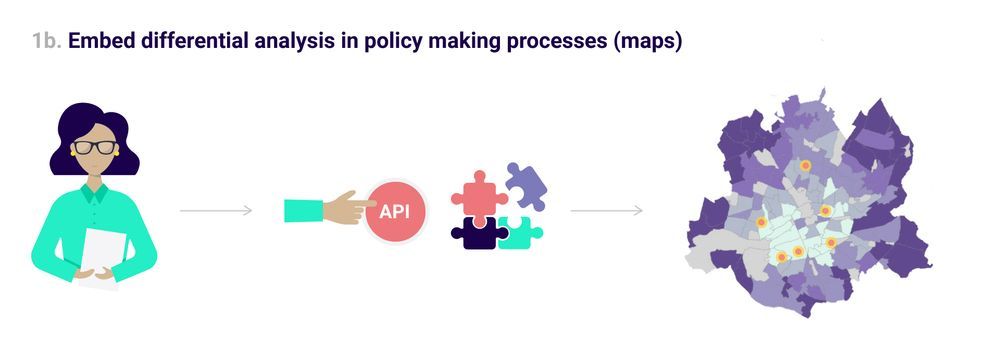
It is hard to even consider these ideas if the disaggregated data is not shown.
2. Measure impacts by disaggregated data
As part of regular reporting, policy heads could create these maps and population reporting mechanisms instantly using the API to keep track of inequitable distribution and potential for structural inequality to be introduced/maintained. That is what we were advocating for as part of the New York COVID-19 population group I am a member of. It is crazy that in this data world, we still do not have these basic tools available.
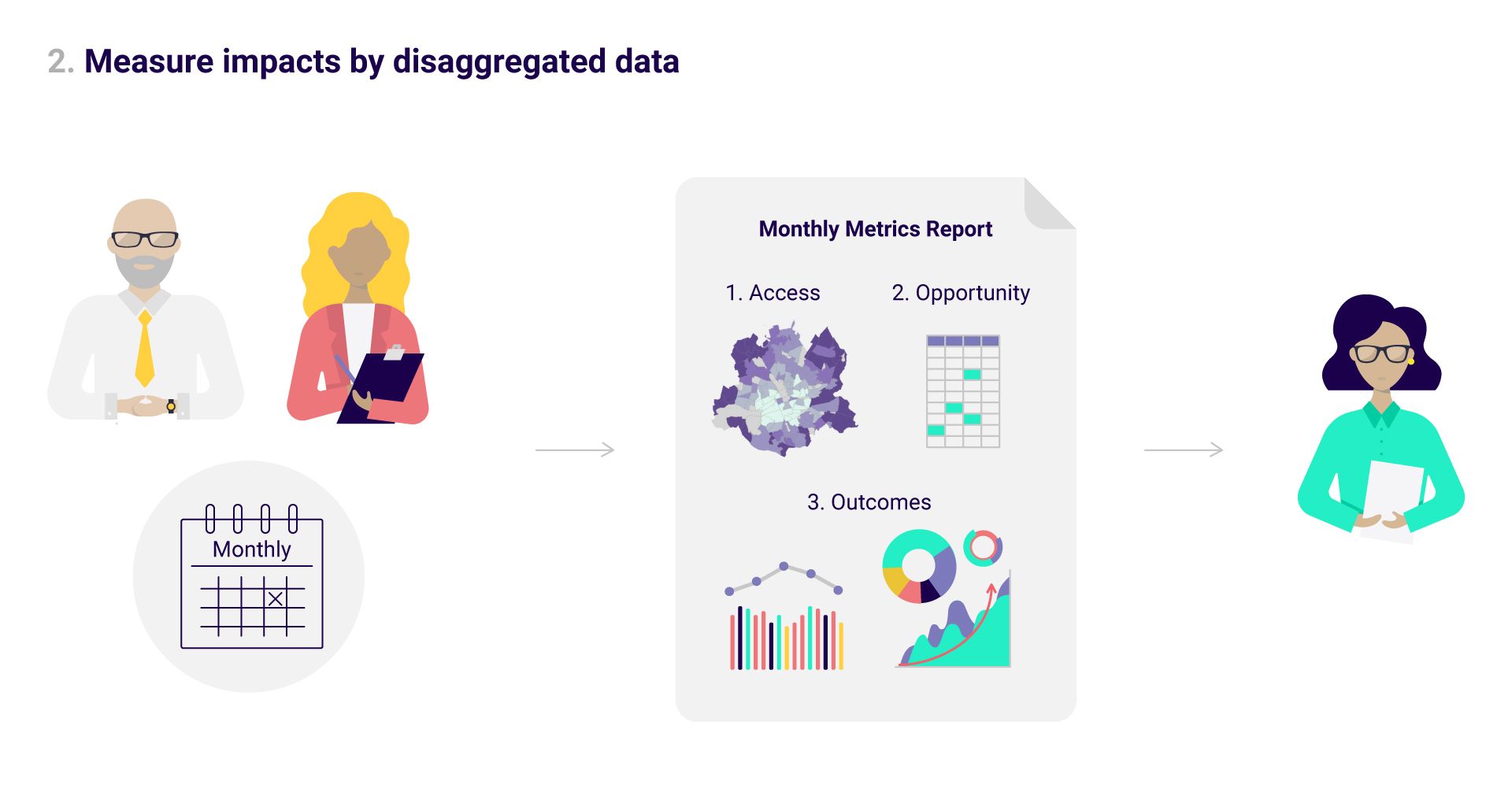
For example, the European CDC uploads a spreadsheet file each day of latest COVID-19 trend data (none of it disaggregated). That means anyone creating a report or a dashboard would need to go to the site, check if a new spreadsheet has been released, download it, clean it so it matches their input needs, and then upload it to create the new report or dashboard refresh. If this was a disaggregated data API, hundreds of stakeholders (researchers, community groups, member state health and policy makers, media) could all integrate the API and have real-time dashboards and reports that showed the data. From there you can then do automated processes like setting up alerts so that automatically a government office is informed if PPE supplies in a particular region drop below a certain threshold, or if tests spike in a given area. If this was disaggregated data via API, you could create a mix of universal and targeted strategies so that those communities most at risk of negative impacts will receive support.
Disaggregated data is not enough: Other API-related processes to address structural inequality
While disaggregated data can be made available via APIs so that equity-based policy can be developed and monitored, it is not enough in itself to help reduce inequality. Government technology and policy teams should be more diverse. This will help nonwhite, non-heterosexual male perspectives to be incorporated into API decision-making activities within government. (Disaggregated data can help monitor the diversity of teams).
Other strategies that can also help ensure APIs include diverse ecosystem participation and conducting impact assessments when commencing government API activities.
3. Conduct impact assessments during government API design activities
Unfortunately, there are not a lot of current tools available for Government API designers to use to conduct impact assessments of government APIs during the design phase. The disaggregated data is an important place to start. Our Framework suggests looking at the European Commission’s Better Regulation Toolbox (specifically tool 13) and adapting that to API discussions. Having worked in local government in the past, I know that impact assessment processes are often seen as onerous, detailed activities that teams are not resourced to complete. They are often saved for large-scale environmental or land use planning projects where there is a legal requirement and this is often outsourced to the developers conducting the projects.
My fear is that if we suggest impact assessments for government API projects that it will be seen as an obstacle for action, and if you look at the Better Regulation Toolbox, then it is a long series of checklists that can feel overwhelming. And again, with the lack of available research, government API teams are stuck with being able to source materials that can help analyse previous equity results to consider in their current work. (Samantha Roberts from the City of Eugene, Oregon, describes a short and long-form Triple Bottom Line process that could be useful as an impact assessment type of series of questioning for those who want to overwhelm their colleagues from a full impact assessment process.)
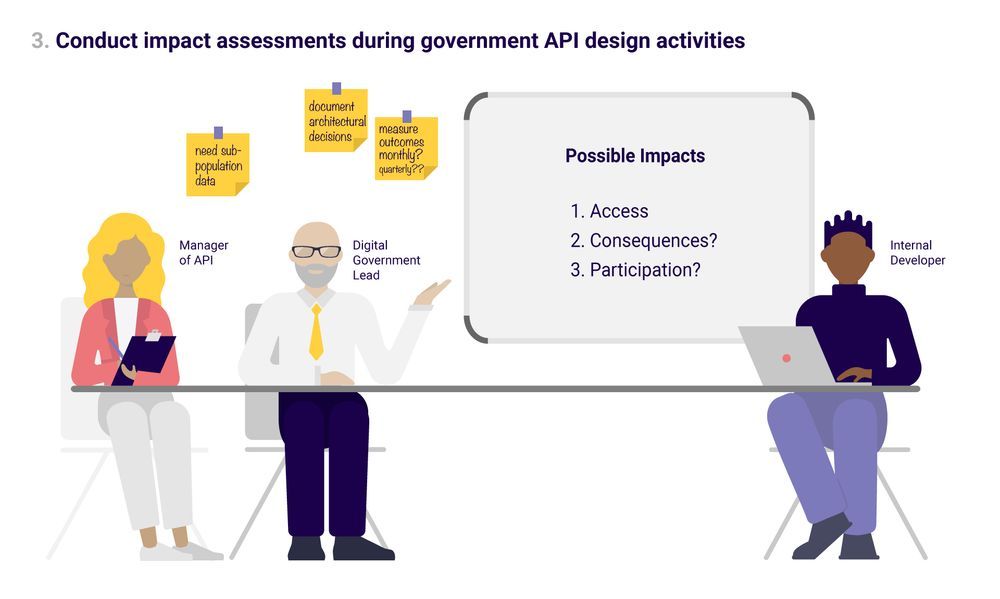
If you have a diverse team, and are speaking with diverse external ecosystem participants (see strategy 4 below), the idea is that you could do a whiteboard type exercise of potential equity impacts and factor these into the API design.
So for example, if an API team is considering introducing an Open311 API for their city, they may brainstorm what could be the potential equity impacts of their implementation. Maybe they come up with the issue of the inadvertent increased resourcing of higher income neighborhoods if that is where the calls come from. So as part of their API design, they recognise the importance of doing disaggregated data collection on a regular basis to monitor this risk. Maybe they invite community groups or the local representative from the low income neighborhood to the ecosystem meetings to advise. Maybe they make an API that feeds sensor data directly into the Open311 (for example, fleet vehicles could have a sensor that sends an alert whenever the vehicle drives over a pothole, or noise alert sensors could send data from a neighborhood whenever a noise threshold is exceeded). In this way, citizens in low income areas would not be required to make the Open311 call in order for their issues to be included. (And suddenly government Open311 API developers are excited about this notion of a sentient/responsive city and start considering even more ideas.)
This will take new skills and creative problem-solving, and again the availability of disaggregated data will be helpful as a jumping off point for teams to use when thinking through the potential impacts on various sub-populations. My experience is that at present, this discussion does not occur when government teams start building APIs.
Nadia Owusu, Associate Director at Living Cities, recommends government teams ask four key questions on differential impacts of outcomes for communities of colour. These questions can also be asked of impacts on people with disabilities, migrant citizens, low income households and LGBT populations. I believe it is worth asking about each of those groups individually, not just saying “marginalised groups” as a catchall. By specifically considering impacts on each of these sub-populations, teams have a greater likelihood of identifying real impacts that need to be addressed in government API activities. You can see the original questions on Quartz, I have adapted them here for Government API impact assessment discussions:
- How has usage and availability of our APIs left out or harmed communities of color in the past?
- Reflecting on that past, what potential adverse impacts or unintended consequences on communities of color could result from our current, and future API activities and their usage by third parties—and how might we work to address them?
- Have stakeholders from communities of color been meaningfully involved and authentically represented in the development of our API activities?
- How might we work to ensure that communities of color benefit from our APIs, and how might we maximize those benefits?
4. Foster diverse ecosystem participation
In the Digital Government API Framework, 12 proposals are divided across three horizontal layers of government implementation and divided into four vertical pillars of activity areas. On the horizontal level, activities are divided into:
- Strategic (a whole of government, policy/strategy level)
- Tactical (a departmental level responsible for allocating resources to implement activities), and
- Operational (the implementation level at which activities are undertaken).
In addition, activities were divided into four pillars:
- Policy support (activities mainly aligned with prioritising and measuring actions)
- Platforms and ecosystems (work to create the collaborative infrastructure needed to deliver APIs across government and with external stakeholders)
- People (the organisational structures that encourage collaboration and skills development)
- Processes (applying best practices).
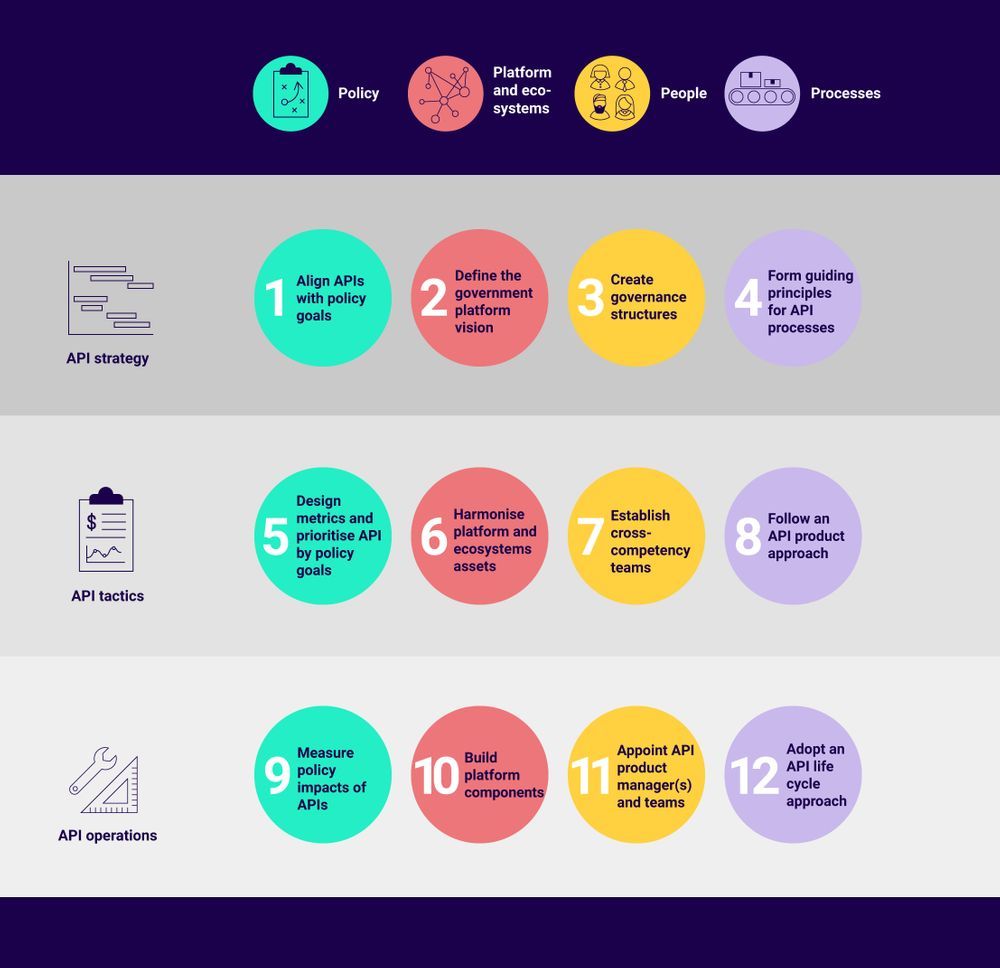
Within the platforms and ecosystems pillar, it is recognised that first, the API team must understand the government’s platform vision (that is, their policy). Some governments decide, for example, to focus on a platform approach in which each department can connect with each other and share common services. Others have a more ambitious view in which governments work collaboratively with external stakeholders to make data and services APIs available to third parties and those third parties then build new products. For example, if a health department were to release government-funded diabetes research data in an API format, third parties could build more apps and services for citizens than the government health department could do on their own, and perhaps those third parties would target specific populations.
Let’s imagine an API team that works in a government that has a platform view of involving external stakeholders. The Framework proposals also recommend taking a user-centred approach. A user-centred approach would ask, ok, if we release a diabetes research data API, what are all the groups that might use that API in their apps? You might think of:
- Supermarkets that want to rate grocery products in their mobile shopping apps,
- Traditional health services and doctor groups, and
- Targeted health services such as a disability advocacy group, Black health agencies in the U.S., health services targeting low income or the unemployed, women’s health services, or a migrant health service provider.
So an equity-based approach to government API development here would be to ensure that when a government is facilitating an ecosystem, that groups that represent those marginalised or sub-populations are represented. Are targeted health services encouraged to participate? In addition, are women-led entrepreneur groups invited? Can small and freelance developers participate alongside enterprises?
As governments release APIs, they also need to use disaggregated data to measure who is using their APIs, and then, where there is under-representation of Black, migrant and women-owned businesses as API consumers, governments need to invest in redressing this balance. As Eric Whitmore notes:
(Eric works at Forward Cities, which is lead by President Fay Horwitt, who has started an amazing series on rebuilding entrepreneurial ecosystems for a more balanced, equitable, sustainable and inclusive future.)
Let’s discuss how Government APIs can address structural racism and inequality
Thanks for reading all the way through. I think this is an important topic that has not been covered in the literature and while I could have written this as a more formal piece, my experience is that a dry, more traditional policy narrative document would not engage with the technologists and API practitioners I am hoping have stuck with me to read all of this. I will write further pieces on this topic for other audiences that do take more of a formal, referenced tone.
If you work in Government APIs, I implore you to consider the four immediate steps that you can take:
- Create population data APIs that can enable click-of-a-button integrations into mapping and writing documents so that we can publish and visualize differential policy impacts by sub-population characteristics. The European Commission’s Open data and Public Sector Information Directive should recognise this as a high value dataset and prioritise creation of an API for this data as an immediate concern. Government statistics departments around the globe can prioritise creating disaggregated population data APIs and then move on to creating tools that can help integrate these APIs into mapping and visualization software.
- Government API teams can then also use these data APIs to report on the uptake of digital government initiatives, including using this data to start measuring who benefits from the APIs they create, and from the apps developed using their APIs. Who uses route planner and weather APIs? Who uses national identity verification APIs? Are women, Black and migrant-owned businesses creating apps and new businesses using government APIs?
- Invite diverse voices to participate in government API ecosystem initiatives. Governments can hold roundtables and ecosystem network meetings to encourage use of government APIs. Make sure nonprofits, small business, women-owned businesses and organisations that represent migrant, Black, LGBT and disability agencies are invited to participate and supported to create value for their communities by using government APIs.
- Brainstorm the potential equity impacts of government APIs. Platformable will be creating a range of tools to help government API teams consider equity impacts during the design phase. The implementation notes in Proposal 1 of the API Framework produced by the European Commission’s Joint Research Centre describes a process and includes a maturity checklist that can help government API teams get started. Reach out or discuss with me in the APIs 4 Digital Government group on LinkedIn to discuss these processes further.
Be willing to use some of your political capital internally to ensure equity discussions are included in Government API design and delivery work. Ask if disaggregated analysis has been done to see who benefits for digital government and government API work.
Where to next?
I am excited to be publishing this article and will be updating my thoughts on this work. I am keen to build alliances with others keen to work in this area. Book time with me via my Calendly (which, by the way, is a Black- and migrant-owned tech business) so we can collaborate on making this work effective (See the end of this article for a direct embed).
This work can extend beyond government APIs. If you work for a private business that is building APIs, I believe that measuring equity impacts is equally important. By ensuring diverse users of your API, you are strengthening and widening the economic opportunities and viability of your ecosystem. I’ll work on a followup article to demonstrate the business value of fostering diversity in your ecosystem partnerships, but please reach out if you have anything to share.
In the future we will be integrating a comments discussion system into Platformable’s website. In the meantime, our discussions on this topic will be held on LinkedIn, in particular the APIs4DigitalGovernment group. Please join me there.
The Platformable team is also available to work with government API teams who would like to test our impact assessment and equity-focused processes so that they can be incorporated into your API design and digital government projects. Get in touch via email or book a meeting with me directly: